Most Likely to Succeed
Fair is fair... isn’t it?
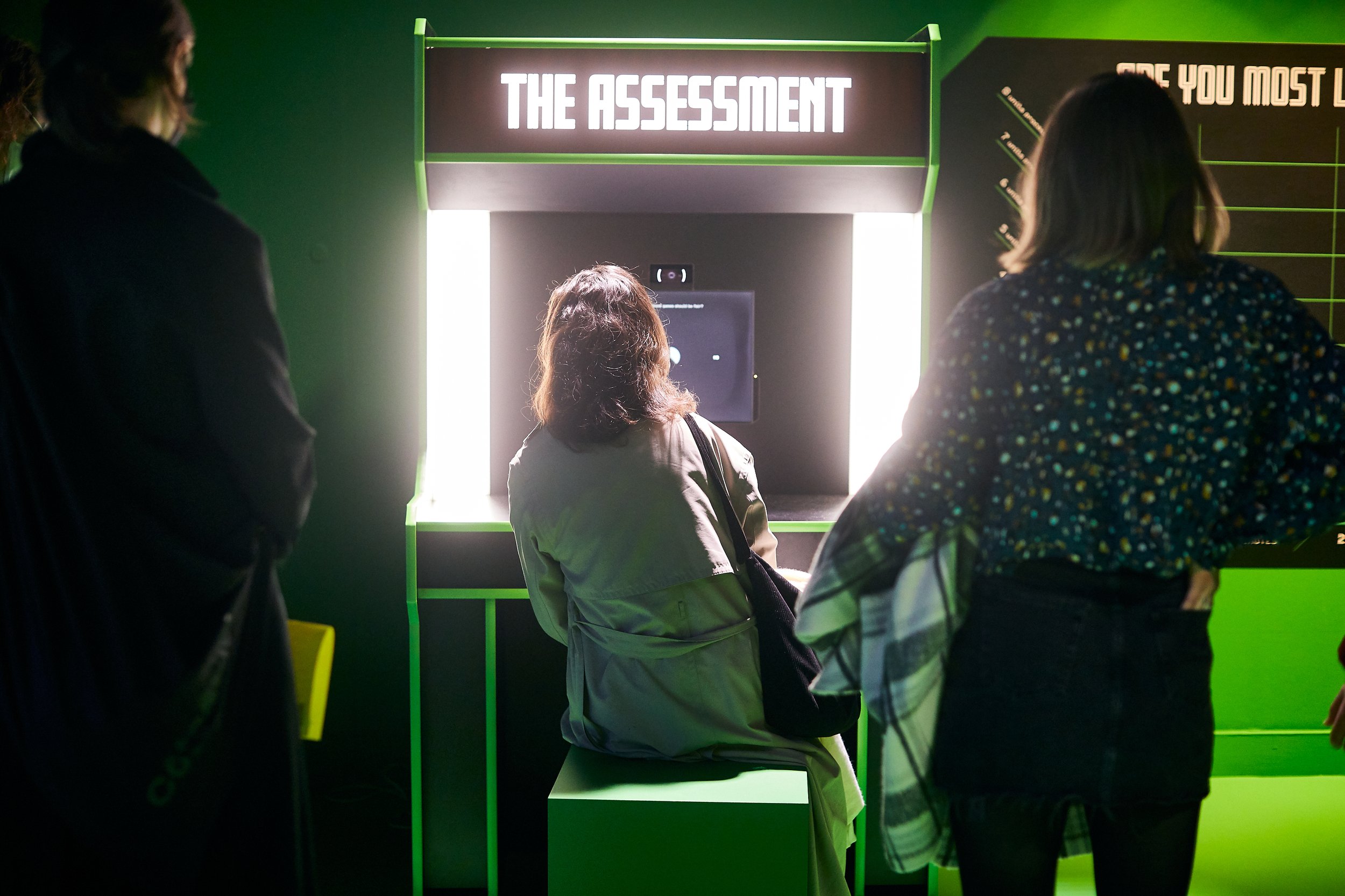
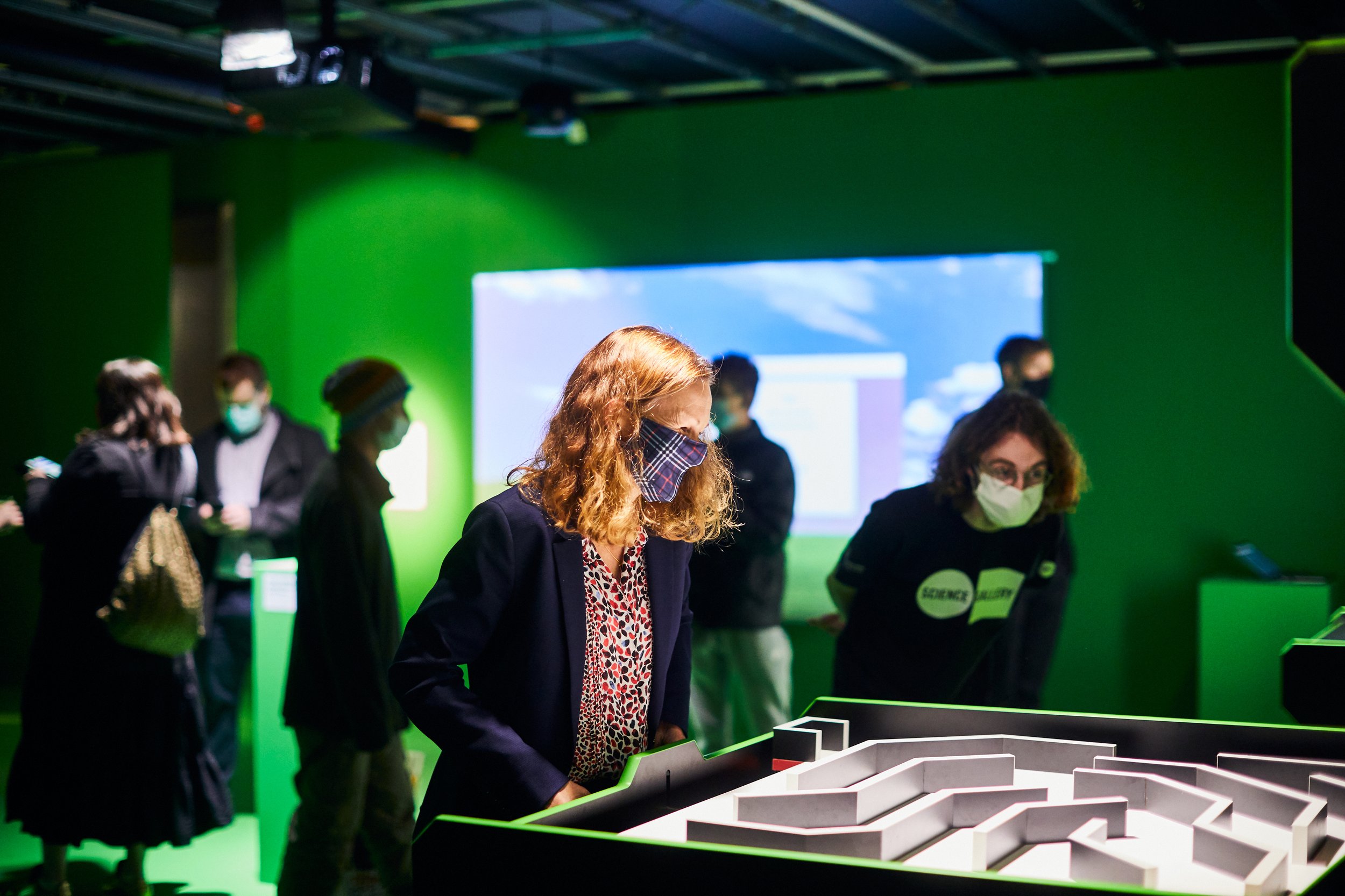
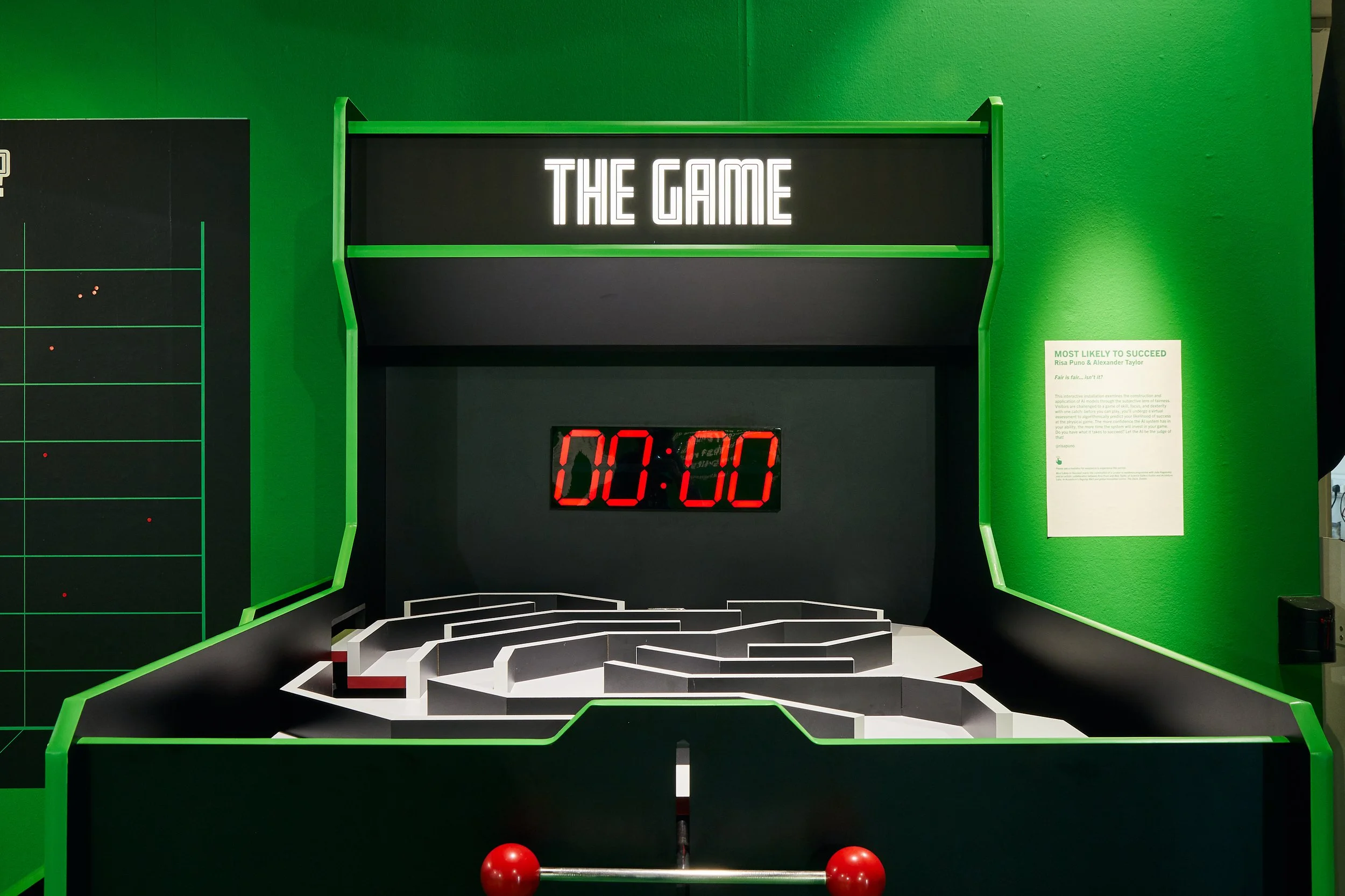
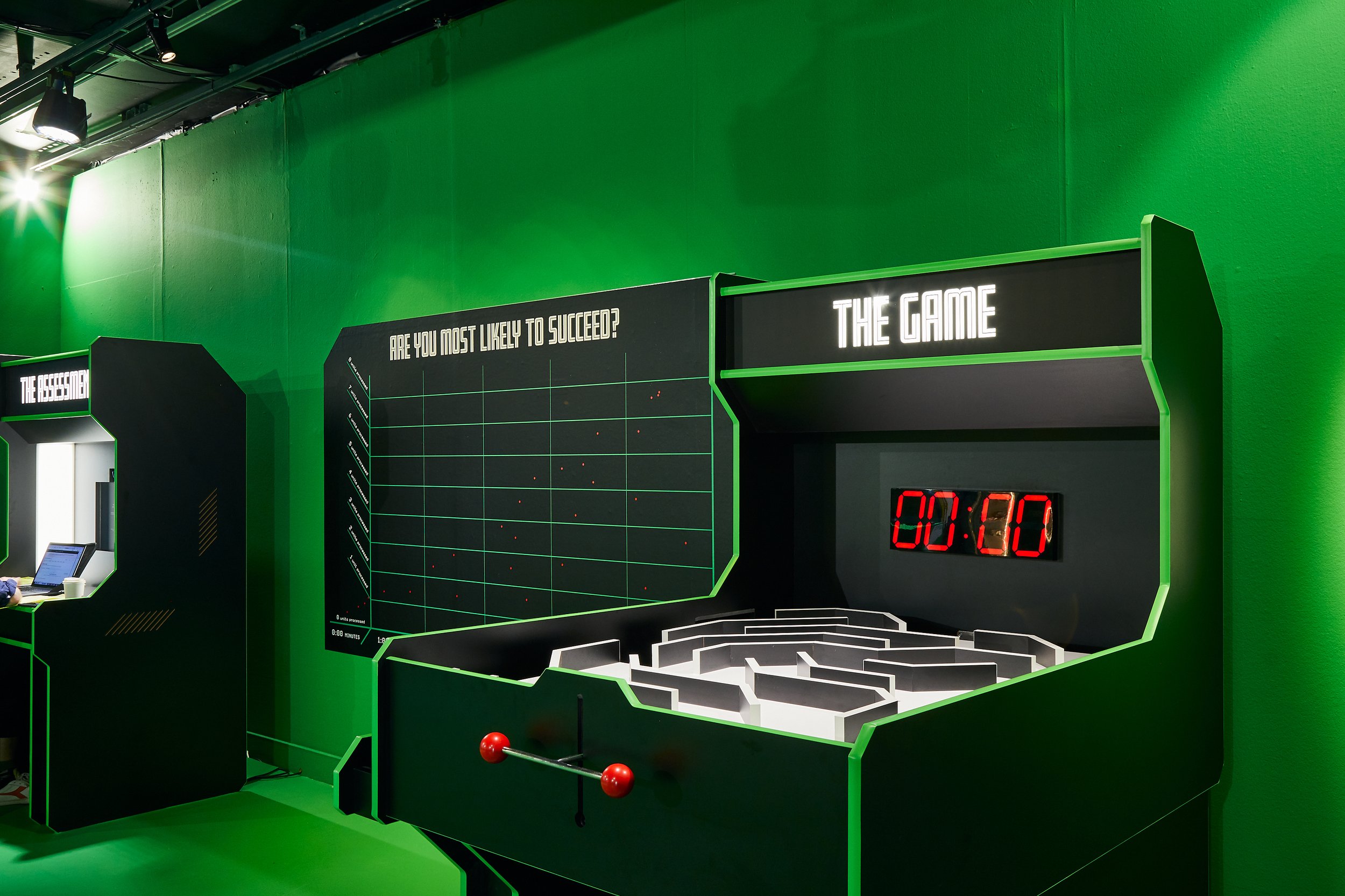
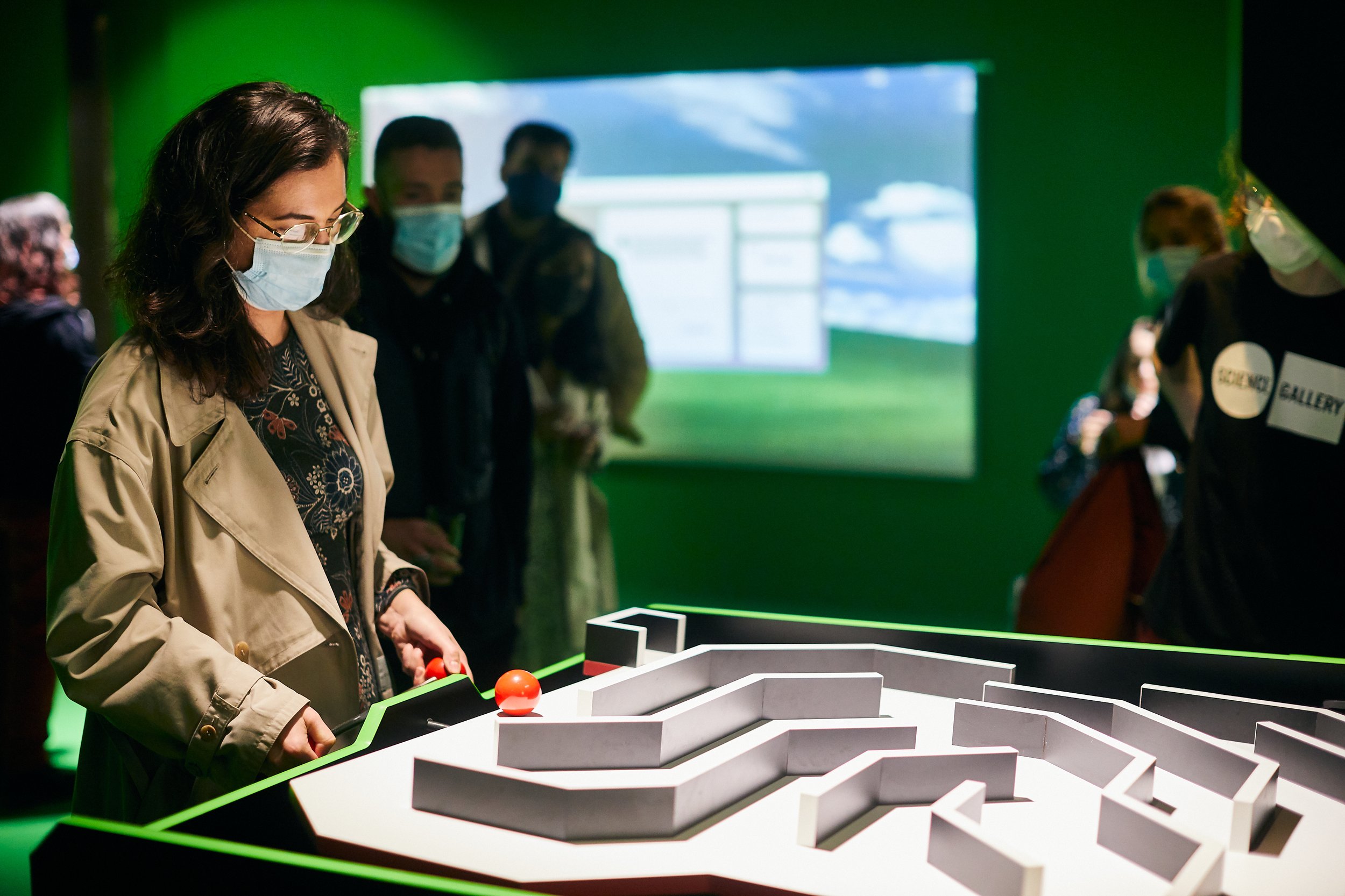
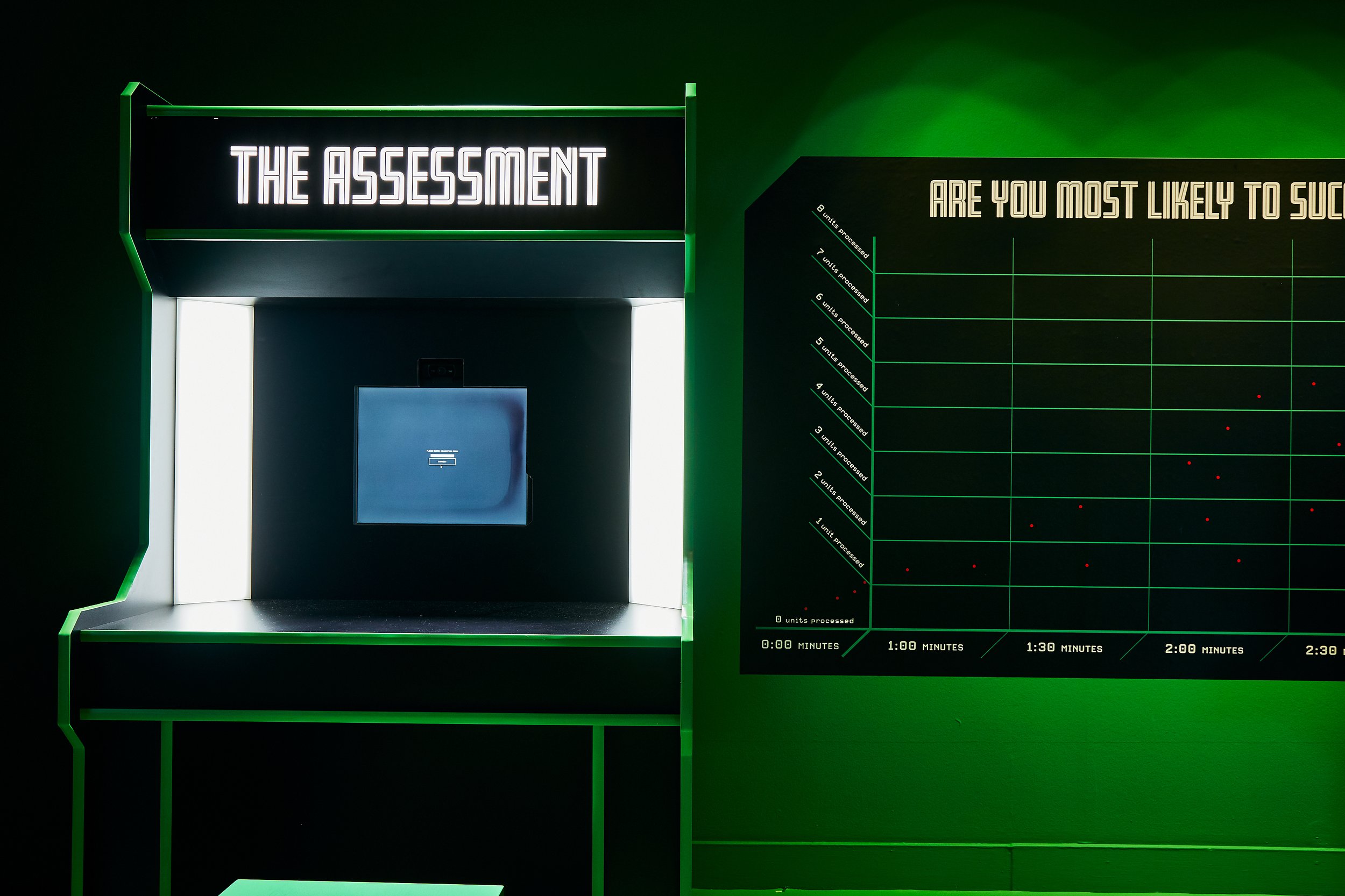
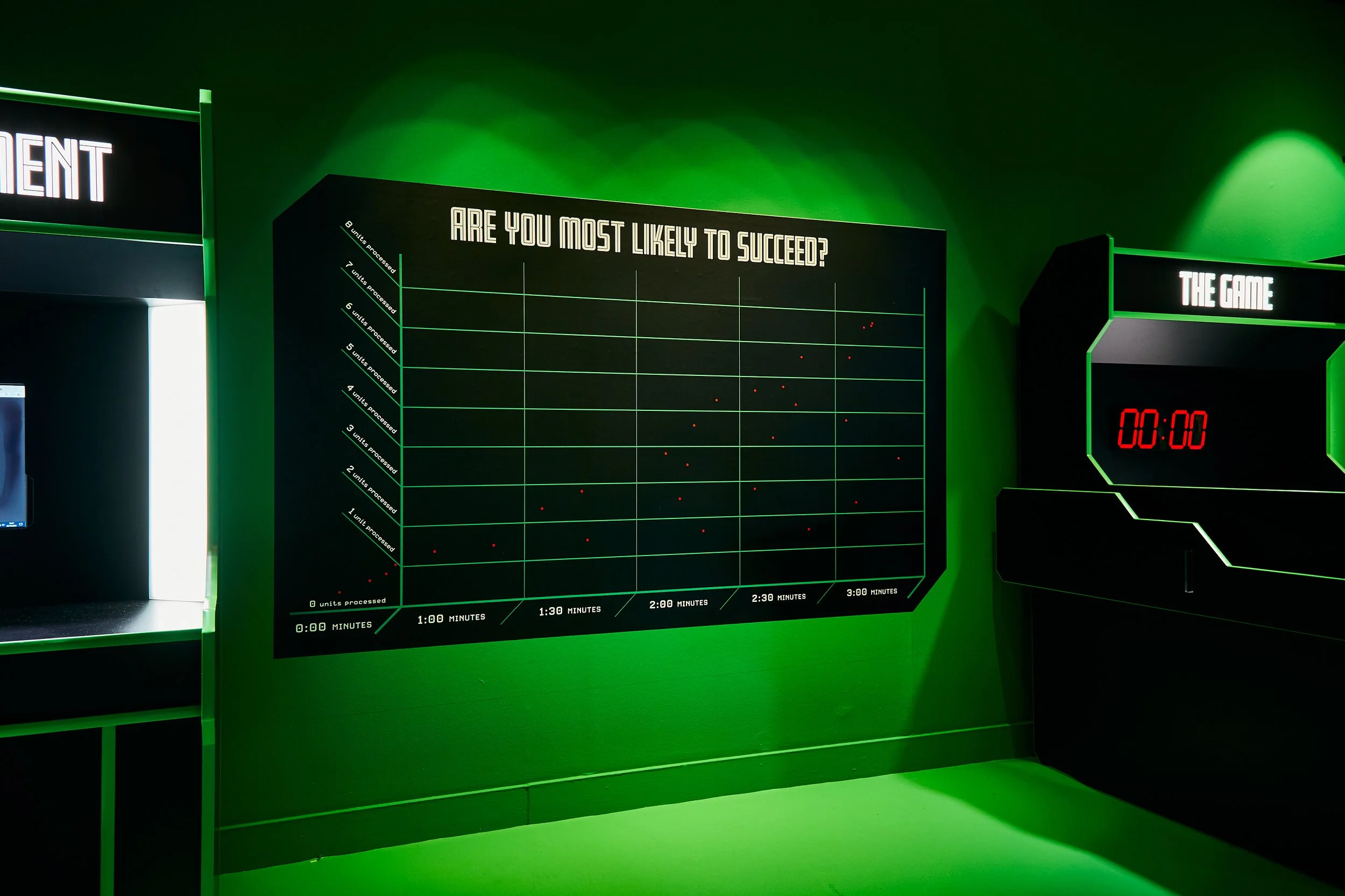
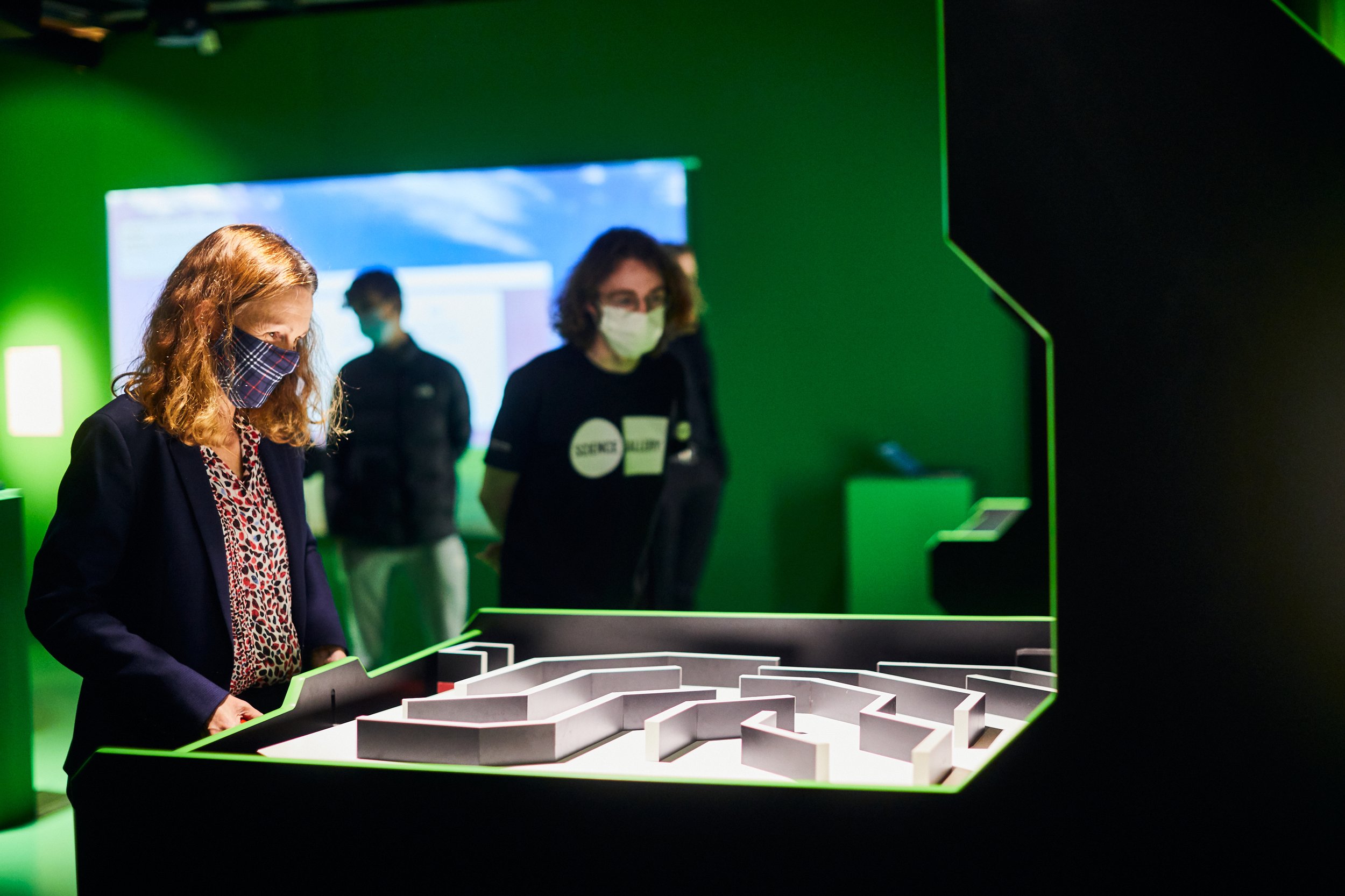
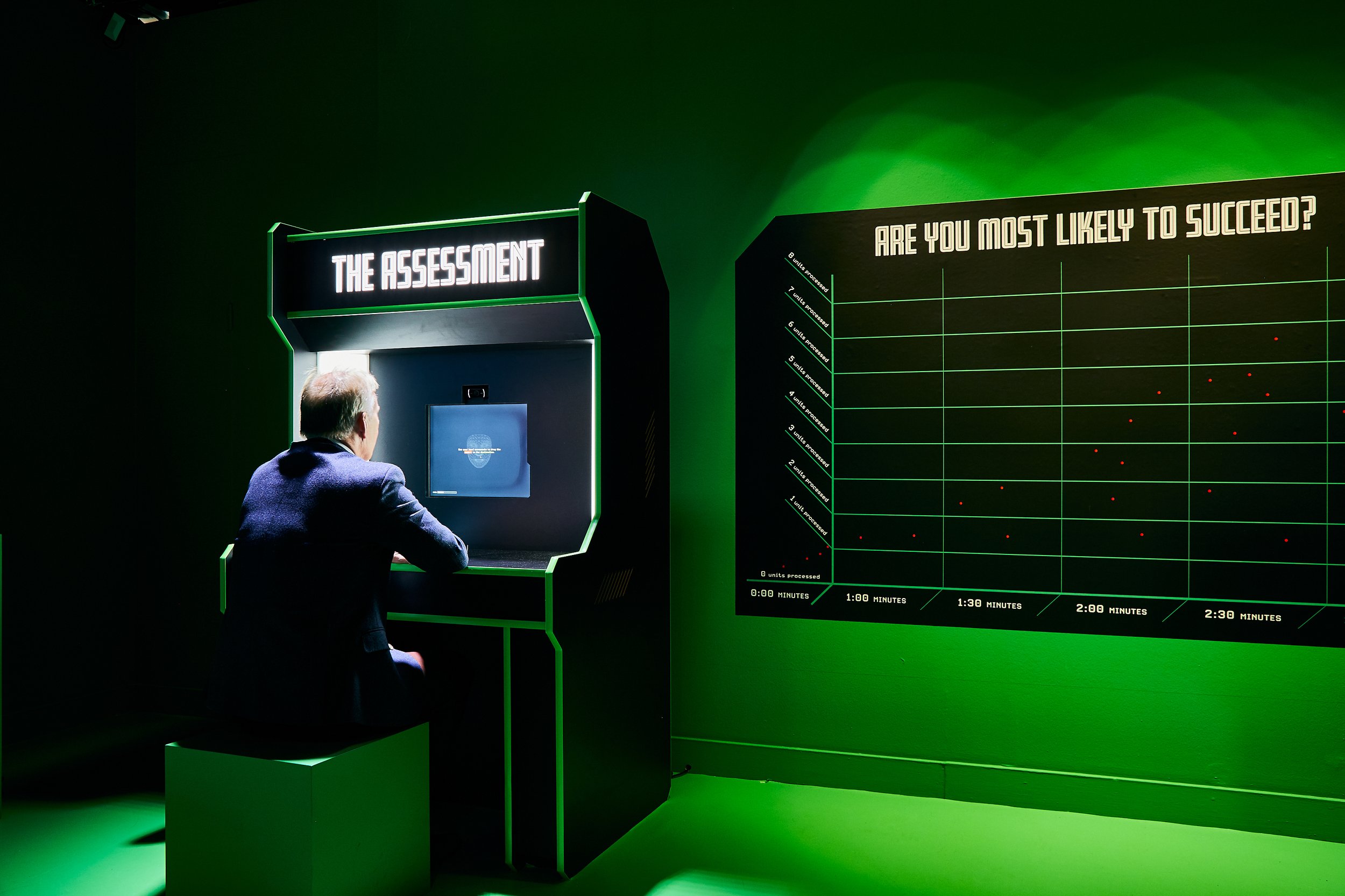
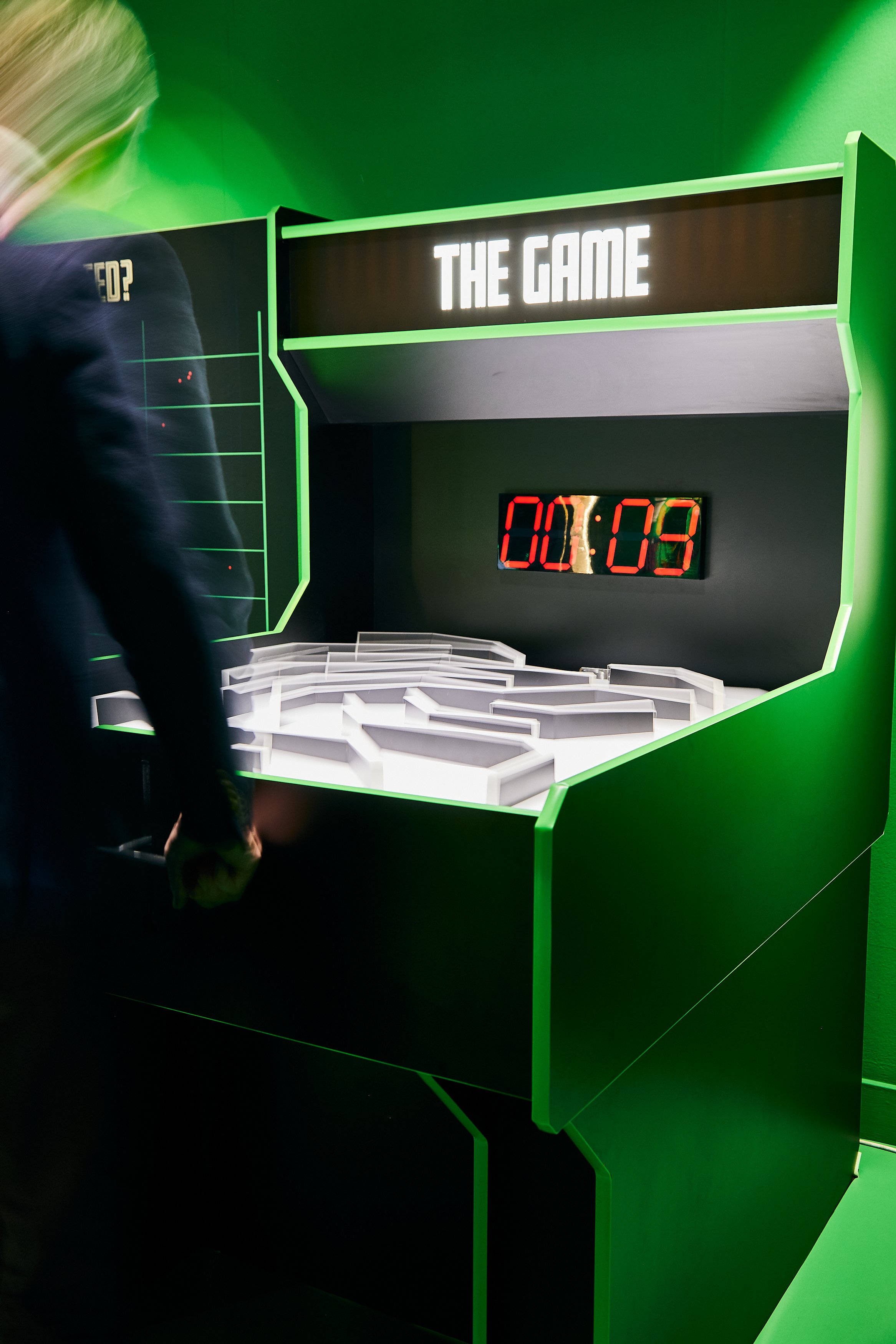
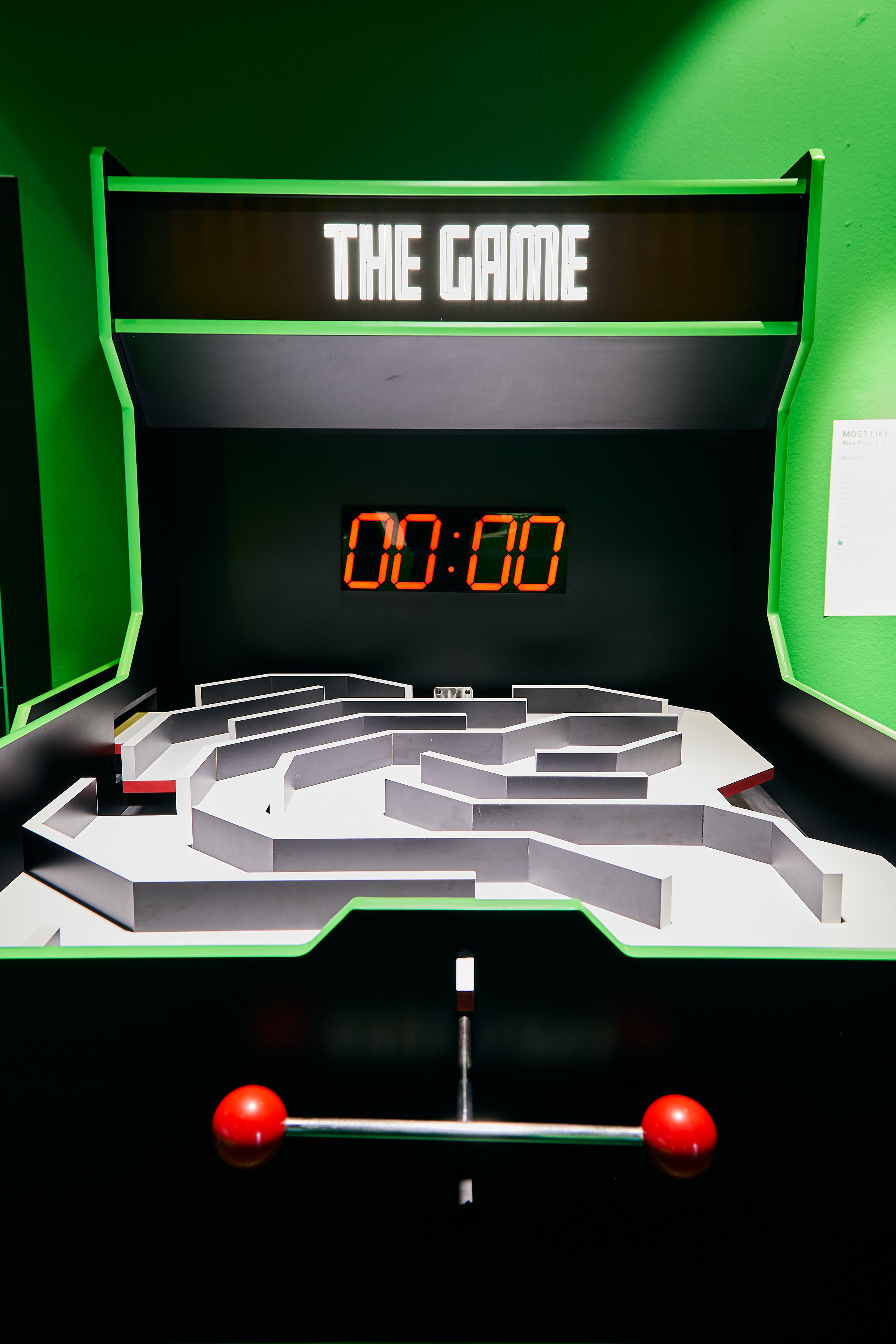
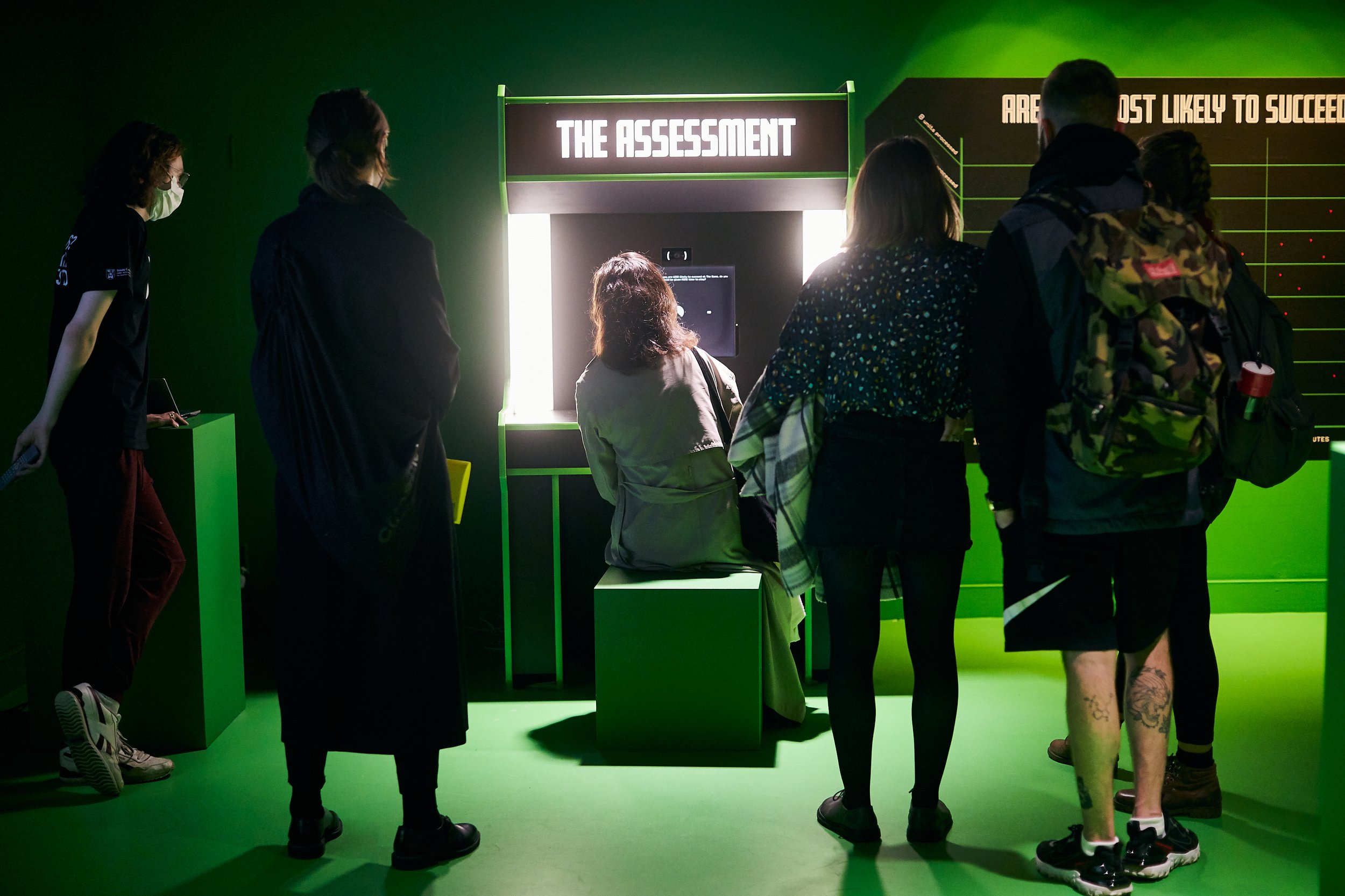
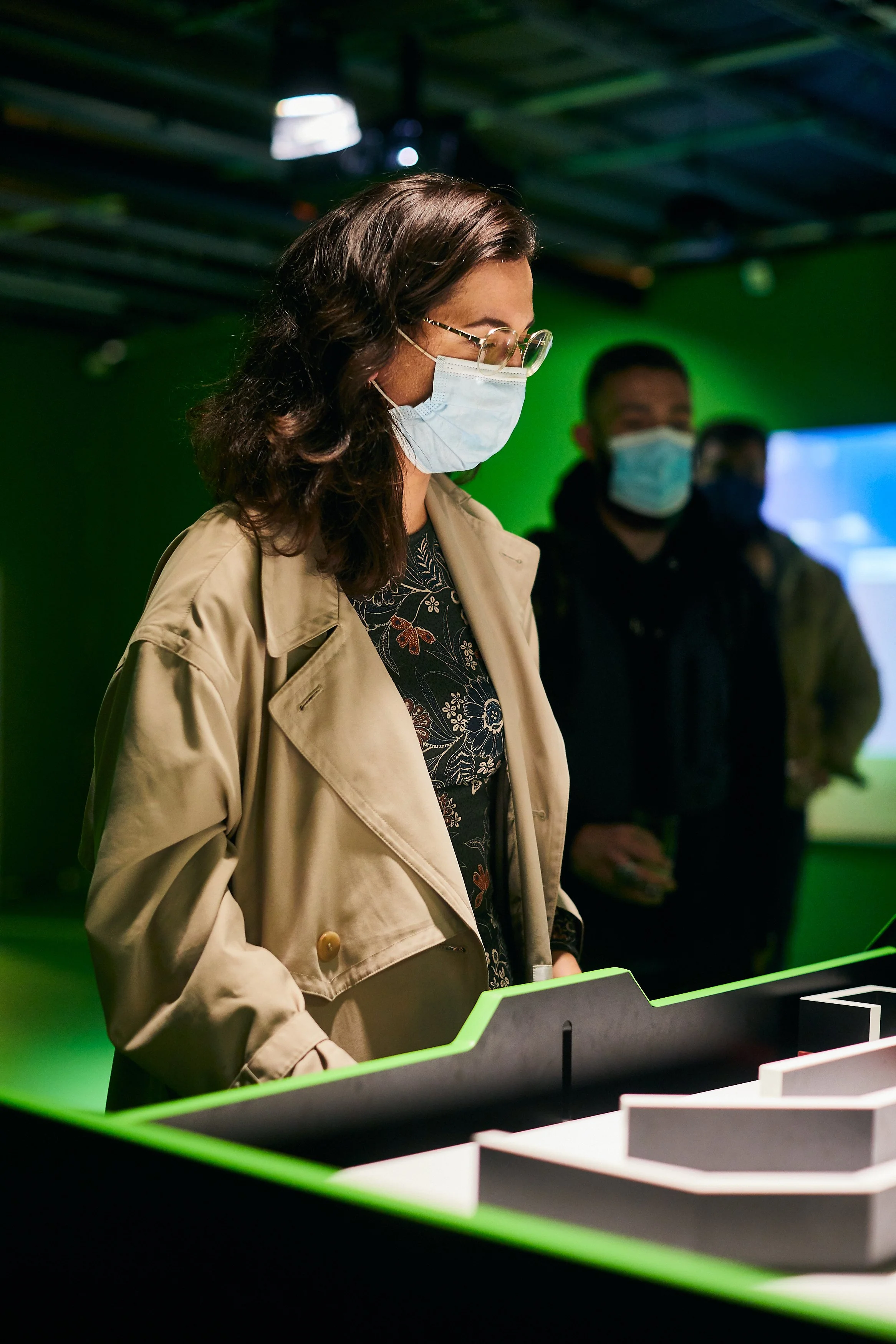
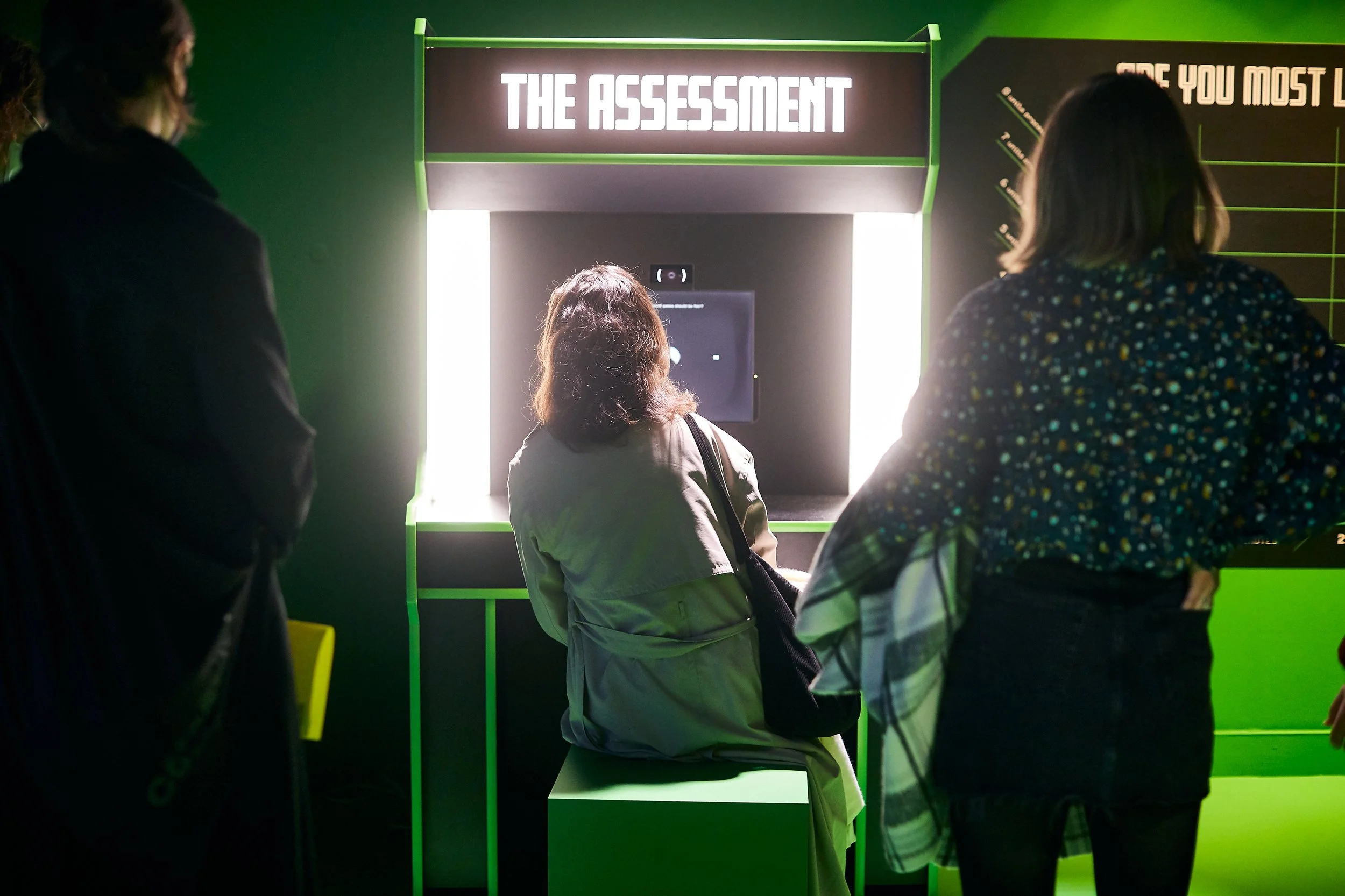
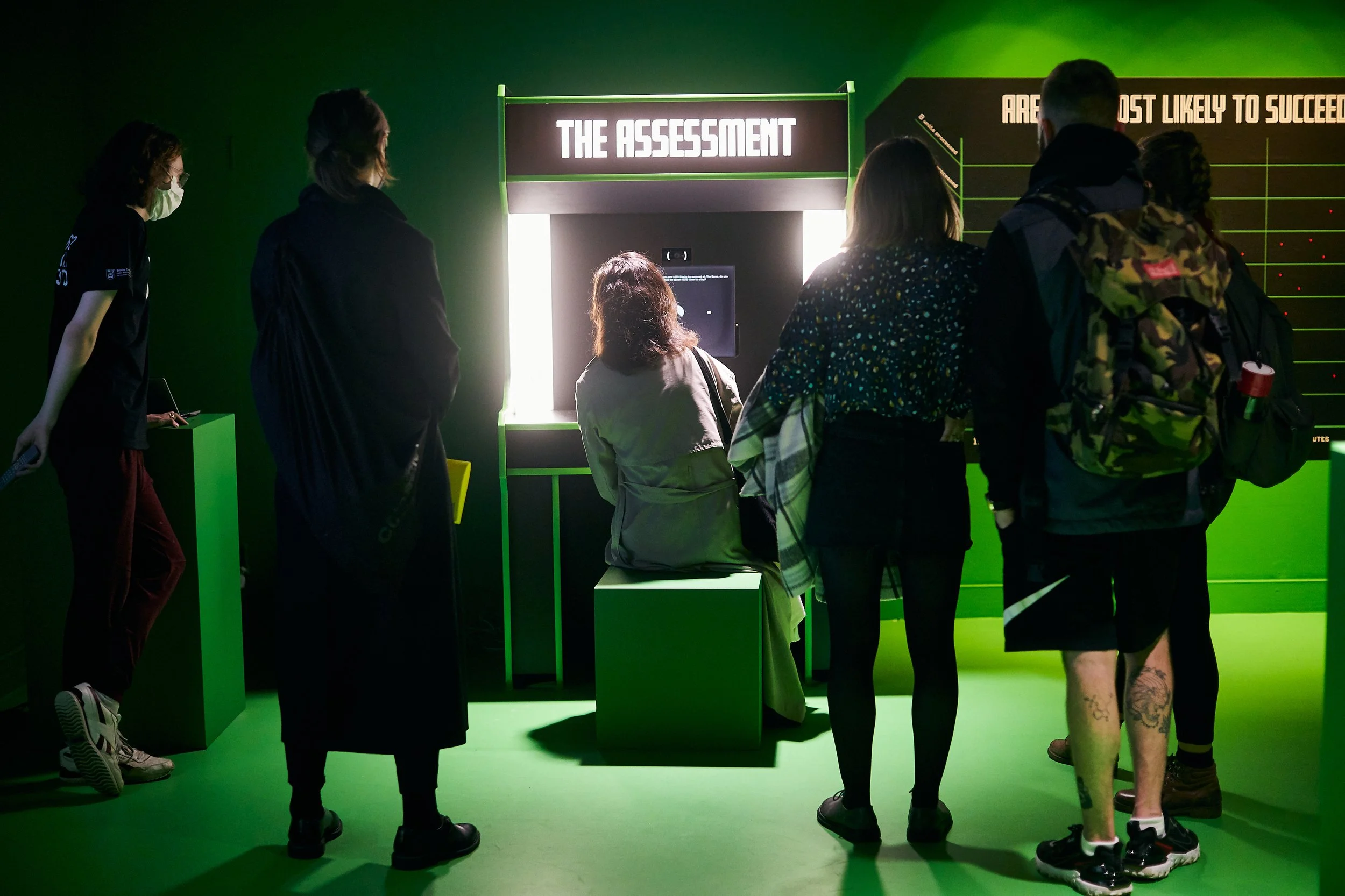
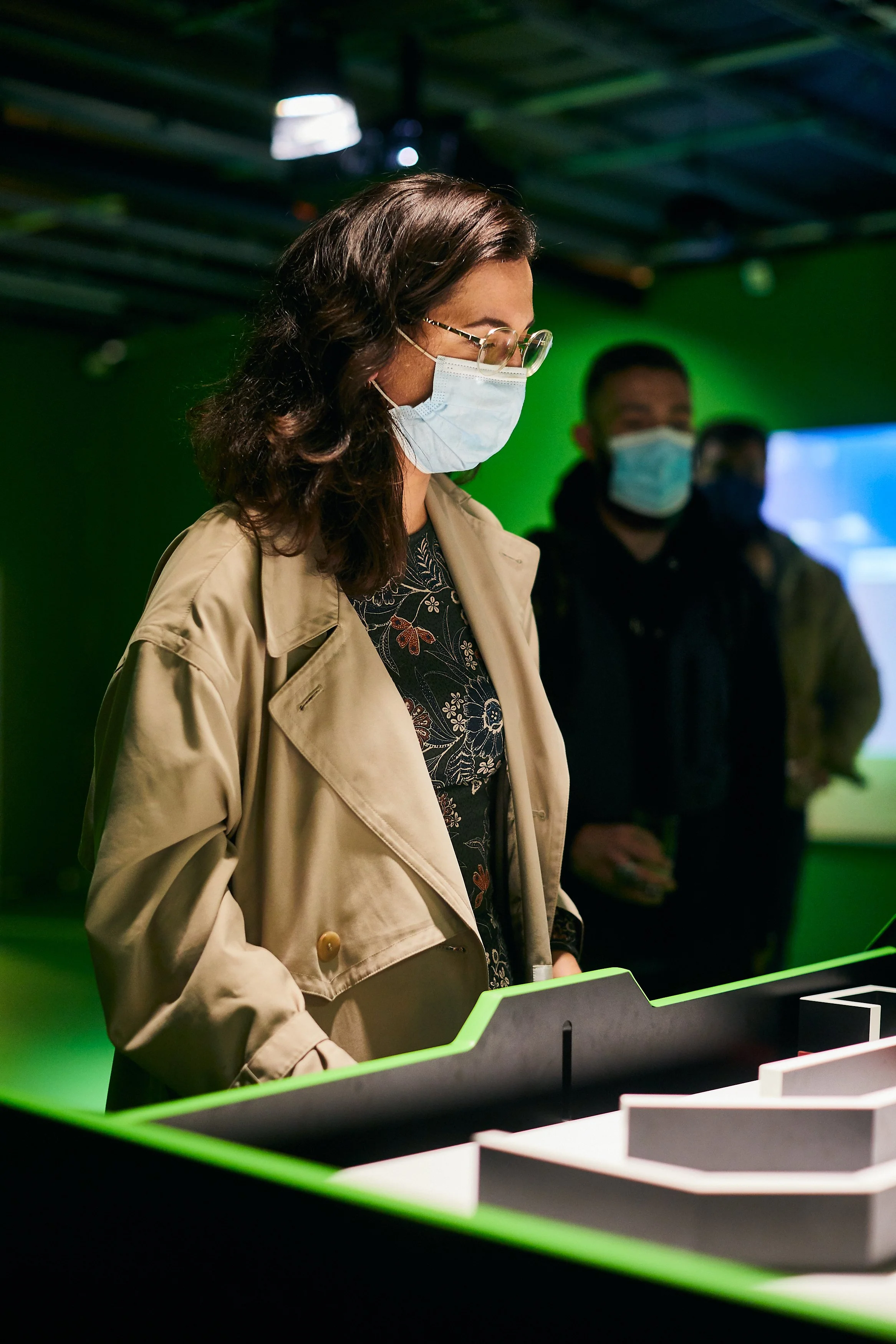
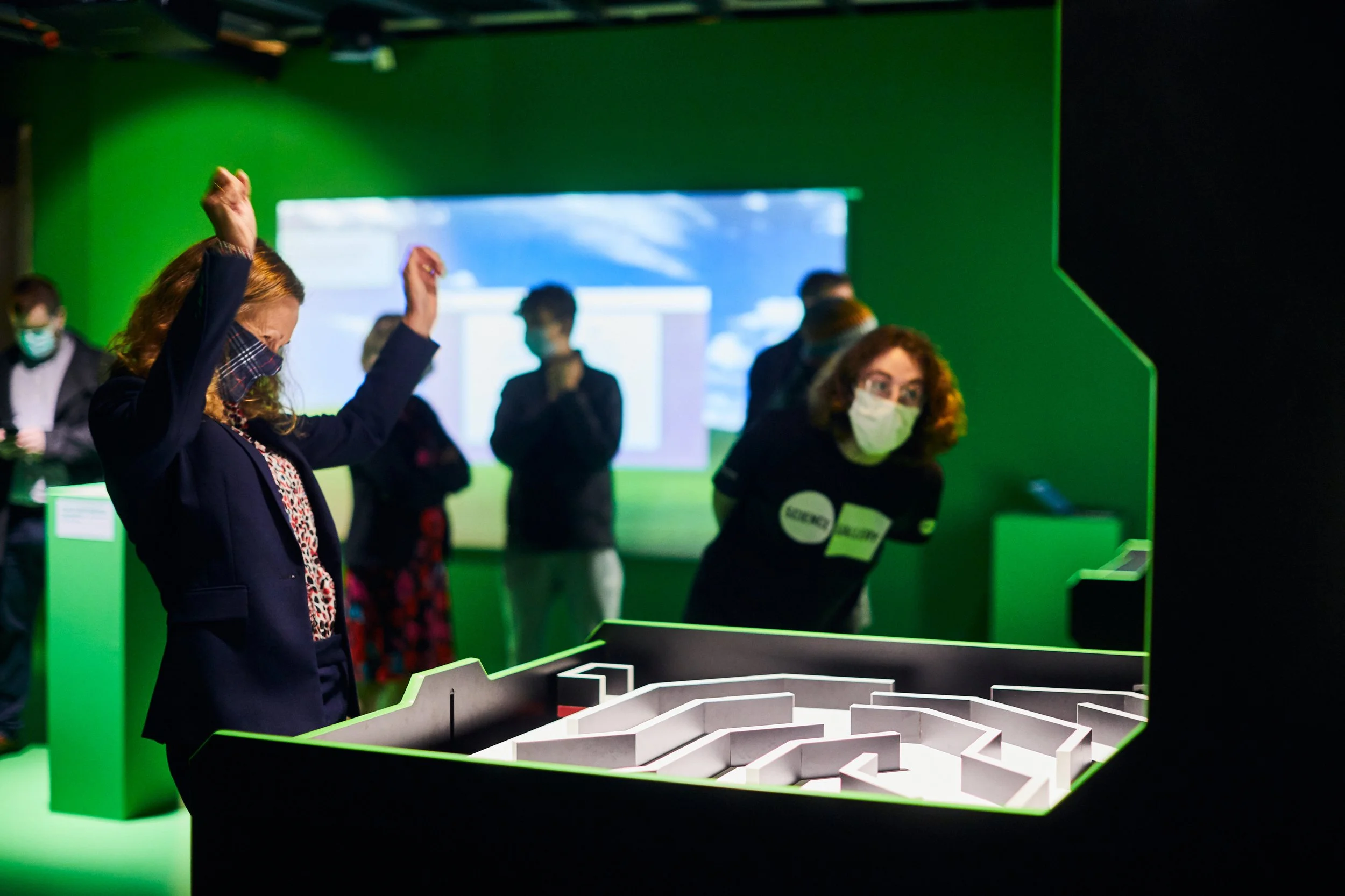
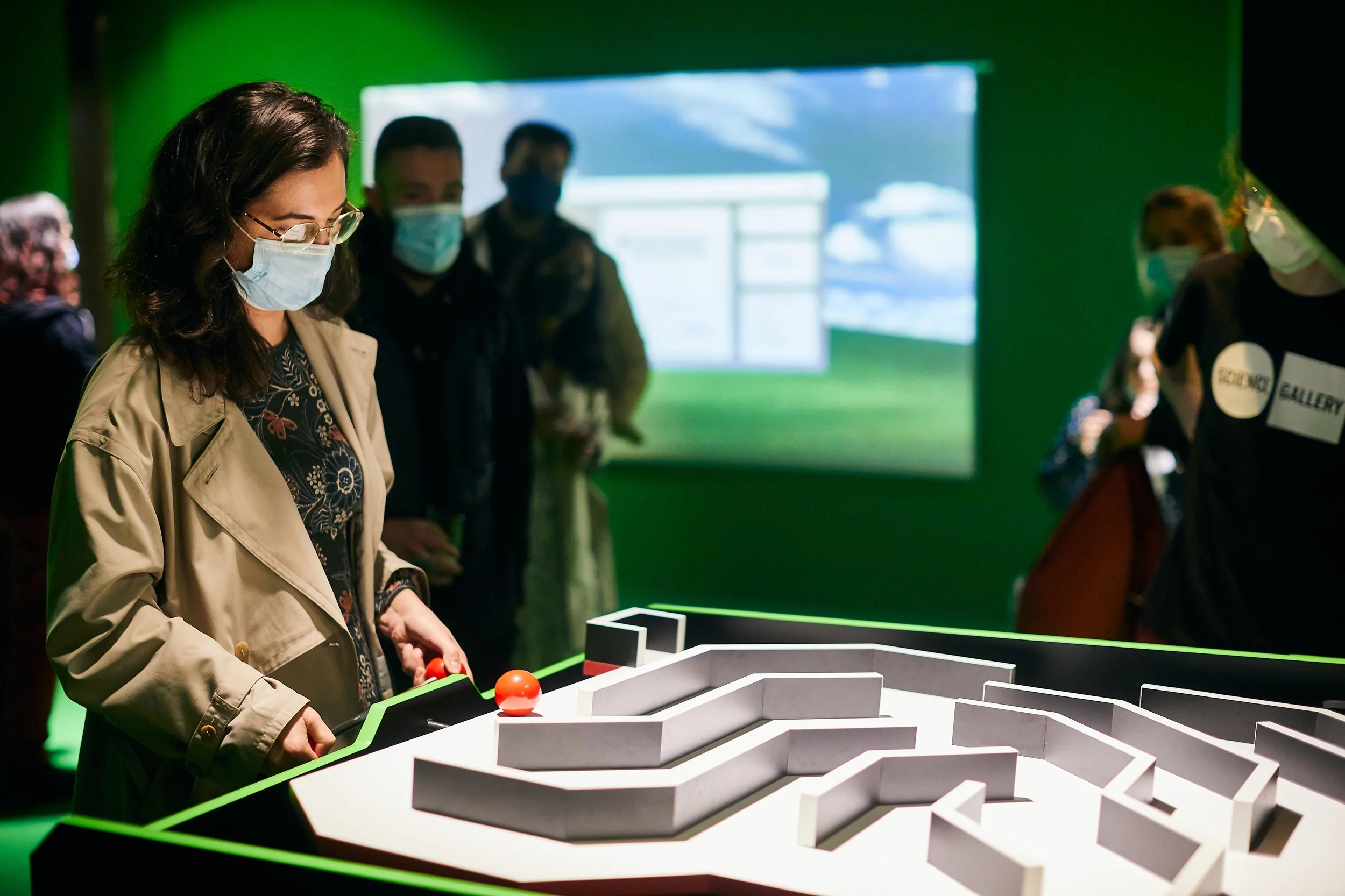
Risa Puno, Alexander Taylor
Most Likely to Succeed is an interactive installation that gives you the chance to prove your skills in an exciting tactile game requiring focus and dexterity. By manipulating a tilting platform, players are tasked with getting as many balls through a maze as possible before time runs out. But there’s a catch: the amount of playing time each person gets is allocated by an AI system. Before playing the game, you must undergo a virtual assessment designed to test skills, such as: reaction time, ability to follow directions, and creative problem solving. Based on your performance, the AI will make a prediction about your likelihood of success in the real-life game. The more confidence the AI system has in your ability, the more time it will invest in your game. Afterward, players receive a performance report that offers insight into how the assessment works and how you could improve your chances and earn a longer playing time. Do you have what it takes to succeed? Let the AI be the judge of that!
AI is increasingly being used to make decisions that impact our lives. From job applications to university admissions to bank loans and insurance claims, machine learning algorithms are making predictions about who will be the best and worst candidate according to a set of criteria that is often hidden away inside a “black box” model. The goal is to increase efficiency and remove the risk of human bias, but how can we ensure that AI systems themselves, and the decisions based on their predictions, are fair? How should we define ‘fairness’ in the first place?
In games, fairness means that all players have an equal opportunity to achieve success. In fact, games actively use mechanics to mitigate potential inequality—for example, flipping a coin to see who gets to make the first move, or handicaps in golf that allow players of different skill levels to compete with one another. However, in real life, there are many conditions and policies that create unfair circumstances. AI systems are introduced with the intent to reduce bias through impartiality, but they are often coded to maximize efficiency and success rates while mitigating risk. Using an AI model that mimics these common real-life standards and applying it to a game, this artwork highlights the potential gap between ‘impartiality’ and ‘fairness.’ It is also designed to encourage visitors to consider which metrics we value and what policies are created based on the resulting model's calculations.
To create Most Likely to Succeed, artists Risa Puno and Alexander Taylor worked with researchers at Accenture Labs to understand the challenges and possible solutions of making AI more fair and less biased. They discussed how fairness could be defined and measured within algorithmic models, as well as how different models can be optimized based on need, equity, or efficiency. They also learned about how researchers attempt to mitigate bias in AI by making these “black box” systems more transparent through Explainable AI, which gives users insight into how the system arrived at its conclusion. At Accenture Labs, researchers are developing a tool called Counterfactual Explanations, which lets the end user know the smallest steps they must take for the AI system to arrive at a different outcome. The artists have incorporated this approach into the ‘report’ that visitors receive at the end of the experience. By showing how a few small changes in your assessment performance could make you appear more likely to succeed in the eyes of the AI, they intend to explore the level of agency this grants the user, as well as the possibilities and limitations of making AI systems more understandable.
Most Likely to Succeed marks the culmination of a curator in residence programme with Julia Kaganskiy and an artistic collaboration between Risa Puno and Alex Taylor, at Science Gallery Dublin and Accenture Labs, in Accenture’s flagship R&D and global innovation centre, The Dock, Dublin.